Published on 07/09/2021 – Last Updated on 07/09/2021 by OTC
The Inverse Synthetic Aperture Radar (ISAR) provides images of objects in rotation concerning the radar. It is based on analysis of the received signal as a function of time and Doppler frequency. The result is a two-dimensional image. Time analysis gives bright points along the radar line of sight axis (range axis), while their position on the cross-range axis is provided by Doppler frequency analysis. It is analogous to conventional Synthetic Aperture Radar (SAR), except that ISAR technology utilizes the movement of the target rather than the emitter to create the synthetic aperture.
Background
As a military reconnaissance tool, SAR, which was developed in the 1950s, was a solution to the 1940s need for an all-weather, 24-hour aerial remote surveillance device. In the late 1940s, the United States Army was looking for an aerial reconnaissance tool that could see through clouds and would not depend on daylight hours. Radar โ given its ability to penetrate clouds and fog and its non-dependence on the wavelengths of visible light โ seemed the logical choice. However, to achieve a high enough resolution to be helpful, the obstacle was that the radar antenna would have needed to have been about the size of a football field, far too large for a reconnaissance aircraft to carry.
Carl Wiley, working at Goodyear, Arizona (which later became Goodyear Aerospace, and eventually Lockheed Martin Corporation) in 1951, suggested the principle that โ because each object in the radar beam has a slightly different speed relative to the antenna โ each object will have its doppler shift. A precise frequency analysis of the radar reflections will thus allow the construction of a detailed image. As a result, a radar antenna a meter or so vast can be made to acquire a photograph that otherwise would require a much larger one.
SAR
It is a primarily coherent airborne or space-borne side-looking radar system that utilizes the platformโs flight path to simulate a large antenna or aperture electronically, generating high-resolution remote sensing imagery. Over time, individual transmit/receive cycles (PRTโs) are completed, with the data from each cycle being stored electronically. The signal processing uses the magnitude and phase of the received signals over successive pulses from elements of a synthetic aperture. After a given number of cycles, the stored data is recombined (considering the Doppler effects inherent in the different transmitters to target geometry in each successive process) to create a high-resolution image of the overflown terrain.
The SAR works similarly to a phased array. Still, contrary to a large number of the parallel antenna elements of a phased array, SAR uses one antenna in time multiplex. The different geometric positions of the antenna elements are the result of the moving platform now. SAR systems are valuable in airborne surveillance applications, allowing for practical 24/7 imaging under all kinds of adverse weather conditions. Currently, surveillance satellites are mostly equipped with SAR sensors.
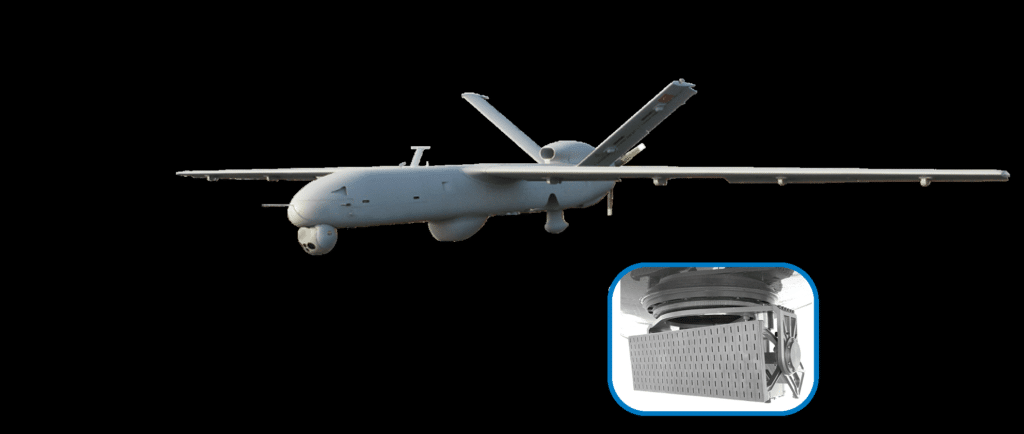
Unlike traditional electro-optical satellite imagery, which can be degraded or denied by adverse lighting conditions or weather, SAR creates images with radar, meaning it can produce images regardless of the weather or lighting conditions. Additionally, SAR sensors can provide data on material properties, moisture content, precise movements, and elevation, meaning that SAR can be used to build 3D recreations of a given geographical area. Itโs planned for U.S. Navy, SAR satellite constellation will collect sub-0.5 meter imagery, capable of identifying various types of aircraft or vehicles at ground level.
Capella Space announced a contract on May 13 to provide airborne SAR imagery and analytics services to the U.S. Navy. Before the COVID-19 pandemic, Capella planned to launch seven satellites in 2020. However, the pandemic has disrupted its supply chain and delayed some launches. As a result, some launches initially scheduled for 2020 are likely to occur in 2021.

ISAR
It is a radar technique using radar imaging to generate a two-dimensional high-resolution image of a target. ISAR radars have a significant role aboard maritime patrol aircraft to provide them with radar images of sufficient quality to be used for target recognition purposes. In situations where other radars display only a single unidentifiable bright moving pixel, the ISAR image is often adequate to discriminate between various missiles, military aircraft, and civilian aircraft.
ISAR assumes that the target exhibits angular motion generally independent of, and typically unknown to, the radar. Thus, it differentiates it from SAR that takes a static target scene. It provides a way to produce high-quality images of ships by use of their angular motion. The main idea is to create an image using the phase difference from the objectโs angular movement. Given the specific nature of the ship movements, using certain radar observation configurations, prints can be produced in the three dimensions of range, bearing, and height. The ISAR antenna can be mounted on an airborne platform. Alternatively, ISAR also can be used by one or more ground-based antennas to generate a 2-D or 3-D radar image of an object moving within the field of view.

ISAR radars have a significant role aboard maritime patrol aircraft to provide them with radar images of sufficient quality to be used for target recognition purposes. Maritime surveillance aircraft commonly use ISAR systems to detect, image, and classify surface ships and other objects in all weather conditions. Because of different radar reflection characteristics of the sea, the hull, superstructure, and masts as the vessel move on the surface of the sea, vessels usually stand out in ISAR images. In addition, there can be enough radar information derived from ship motion, including pitching and rolling, to allow the ISAR operator to manually or automatically determine the type of vessel being observed. For example, the U.S. Navyโs new P-8 Poseidon patrol aircraft carry the AN/APY-10 multi-mode radar system that includes both SAR and ISAR modes of operation.
Visar
DARPAโs Video Synthetic Aperture Radar (ViSAR) program seeks to develop and demonstrate an Extremely High Frequency (EHF) targeting sensor that operates through clouds as effectively as current EO/IR sensors operate clear weather. In addition, the program seeks to develop and demonstrate a cloud-penetrating EHF sensor in a moveable gimbal that could be mounted on a variety of aerial platforms to provide high-resolution, full-motion video for engaging moving ground targets in all weather conditionsโcloudy or clear.
Compact flyable EHF-band exciters, receivers, and medium-power amplifiers should have size, weight, and power characteristics to enable the ViSAR sensor to operate using an existing EO/IR gimbal. The EHF-band scene simulation technology effort seeks to develop a synthetic scene model for generating synthetic raw data sets that simulate realistic EHF-band radar data sets. Advanced algorithms for EHF-band operation aim to create and characterize new algorithms that may be enabled by developing EHF-band SAR, which can provide new sensor capabilities or enable more efficient processing. In 2017 the program conducted flight testing of the ViSAR sensor, which successfully captured real-time video through clouds.
How could machine learning be incorporated into the ISAR?
Recent advances in machine learning have enabled rapid improvements in object detection and target recognition algorithms for visible imagery. ISAR generates a two-dimensional image of a target utilizing the movement of the target rather than the emitter to create the synthetic aperture. Using machine learning can be developed an innovative approach that automatically differentiates between classes of vessels using features learned from training datasets. These training datasets for machine learning are of vital importance. Characterizing the training data requirements in the context of the algorithmic approach proposed is required. It informs how users can easily modify the resulting system to identify new classes of targets.
Check outย Naval Library Appย to learn more about the specifications of ISAR radars.
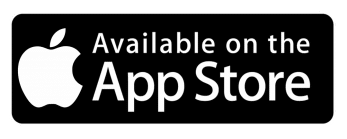

Comments